Data Loss Prevention (DLP) has always been a concern for businesses. In earlier days, the focus was on the protection of physical documents. This could be accomplished by penetrating physical perimeters or theft of documents from couriers.
While these tactics may continue today, the growth of the Internet has increased the magnitude and likelihood of data theft. In short, the proliferation of data and communication channels has made the criminal’s job easier.
DLP has a reputation for being an enormous, multi-year undertaking, yet it need not be. A DLP program can be a manageable, progressive process, if organizations focus on a progressive approach. In the words of Gartner Research VP Anton Chuvakin:
“Deployment of a DLP tool should go from one tactical success to another (a "quick-wins" approach) to avoid outright failure due to complexity and organizational politics.”
A 7 Step Framework for Developing and Deploying Data Loss Prevention Strategy
There are a number of fundamental activities that must occur when initiating a data loss prevention program. This framework provides general guidelines that your DLP strategy should follow. These requirements can also be used to help choose the right DLP solution for your organization.
1. Prioritize data
Not all data is equally critical. The first step in any DLP program is to determine which data would cause the biggest problem were it stolen. Manufacturing companies might choose to prioritize intellectual property such as design documents in their DLP efforts, particularly those for future products. Retailers and financial service companies should obviously rank PCI data highly. Healthcare companies would prioritize medical records (PHI), as these often are stored on laptop computers. While it may seem obvious, data loss prevention should start with the most valuable or sensitive data that is most likely to be targeted by attackers.
2. Categorize (classify) the data
Classifying data is often seen as a formidable challenge in DLP. A simple, scalable approach is to classify by context; associating a classification with the source application, data store, or user who created the data. Applying persistent classification tags to the data allows organizations to track its use. Content inspection, which examines data to identify regular expressions representative of social security and credit card numbers or keywords (e.g., “confidential”), is also useful and often comes with pre-configured rules for PCI, PII and other standards.
3. Understand when data is at risk
This may vary with the type of data. Encryption and network-based security controls may provide security when data is at rest, inside the firewall. For data distributed to user devices, or shared with partners, customers and the supply chain, different risks are present. In these cases, the data is often at highest risk at the moment of use on endpoints. Examples include attaching data to an email or moving it to a removable storage device. A robust data loss prevention program must account for the mobility of data and the moments when data is put at risk.
4. Monitor all data movement
Understanding how data is used and identifying existing behavior that puts data at risk are critically important. Without this knowledge, organizations cannot develop appropriate policies that mitigate risk of data loss while allowing appropriate data use.
Not all data movement represents data loss. However, many actions can increase risk of data loss. Organizations should monitor all data movement to gain visibility into what’s happening to their sensitive data and determine the scope of the issues that their DLP strategy must address.
5. Communicate and develop controls
Monitoring will provide metrics about how data is put at risk. The next step for effective data loss prevention is to work with business line managers to understand why this is happening and create controls for reducing data risk. Data usage controls may be simple at the beginning of a DLP initiative, targeting the most common risky behaviors while generating support from line managers. As the data loss prevention program matures, organizations can develop more granular, fine-tuned controls to mitigate specific risks.
6. Train employees and provide continuous guidance
Once an organization understands the circumstances under which data is moved, user training can often mitigate the risk of accidental data loss by insiders. Employees often don’t recognize that their actions can result in data loss, and will self-correct when educated. Advanced DLP solutions offer user prompting to inform employees of data use that may violate company policy or simply increase risk (in addition to controls to outright block risky data activity). In one study, prompting reduced risky user behavior by 82% in just six months.
7. Roll Out
Getting control of your most critical data is an important first step in data loss prevention, but it’s not the last. Some organizations will repeat these steps with an expanded data set or extend data identification and classification to enable more fine-tuned data controls.
Of course, data loss prevention is an ongoing process, not a single set of steps. By starting with a focused effort to secure a subset of your most critical data, DLP is simpler to implement and manage. A successful pilot will also provide lessons for expanding the program. Over time, a larger percentage of your sensitive information will be included, with minimal disruption to business processes.
5 Myths that are Killing your DLP Strategy
Although the need for data loss prevention has gained visibility among security and compliance communities in recent years, many organizations are still reluctant to adopt DLP programs. Often, this hesitation is based on a misunderstanding of the technology. The following are, in my opinion, the top 5 myths that detract from effective DLP strategy development.
Myth #1 – DLP is not for the faint of heart
A common misperception about DLP is that it requires an enterprise-wide effort to begin; all data must be analyzed, all users must be classified, and all endpoints, servers and gateways included. While many organizations will migrate to comprehensive coverage over time, the most successful deployments start small and focused.
For example, pick a data category that is particularly sensitive, such as design documents. These are easy to identify, as they are created by a fixed set of applications (e.g. CAD applications) and have a defined user group that requires access rights. Include business process owners in the discussion to ensure their understanding and buy-in. As discussed in the data loss prevention framework, you can add another data category once the pilot DLP program is running smoothly.
Myth #2 – My network will choke
Examining the contents of all network traffic to identify sensitive data would obviously cause latency, but repeated content inspection is not a requirement for data loss prevention.
Fortunately, inspecting each data packet as it travels on the network isn’t necessary. Instead, data can be classified as it is created or modified on the endpoint (using contextual classification, content inspection, user classification, or some combination thereof). Once classified, a persistent classification tag is added to the data. Intelligent endpoint agents can read these tags and enforce usage rules based on data classification, user type, the requested action, and other contextual aspects of data activity. This results in better visibility and control, without network latency.
Myth #3 – DLP won’t work outside my network
DLP is simple to understand when you only think about devices inside your network, but many believe it’s not effective outside the network or in virtual environments. In fact, data-centric DLP works everywhere, because the protection is applied to the data itself, not the device, network, or user account.
DLP applied at the data level can automatically prevent sensitive data from leaving your network. It can also force any data that does leave to be encrypted (and decrypted only by devices you manage) or be transferred to approved devices.
Myth #4 – Content analysis is required, and complicated
As discussed, content analysis examines file contents for specific patterns, such as social security and credit card numbers. While this can be useful for PCI and HIPAA compliance, it isn’t a requirement for effective DLP.
Contextual awareness allows for a simpler means of classifying data automatically, simplifying classification and accelerating DLP adoption while preserving the privacy of employee communications. Rather than examining the data content, it associates a classification with the application(s) used to create the data (e.g. financial or design software), the users creating the data (e.g. a member of the legal team), the storage location of the data (e.g. a finance folder on a drive), and other pre-defined characteristics.
Myth #5 – DLP will interfere with legitimate use of data and affect productivity
This myth is a holdover from DLP’s early days, which were plagued by convoluted permissions and ineffective data classification. Modern DLP, applied at the data level, does not affect legitimate users following corporate policies. Endpoint agents classify data automatically and enforce policies transparently. This data loss prevention approach will, if desired, block unauthorized use, but it can also be used in non-obtrusive ways, such as warning or prompting users about risky behavior. This capability reinforces an organization’s security policies, and provides timely guidance that allows users to self-correct habits that put data at risk of loss.
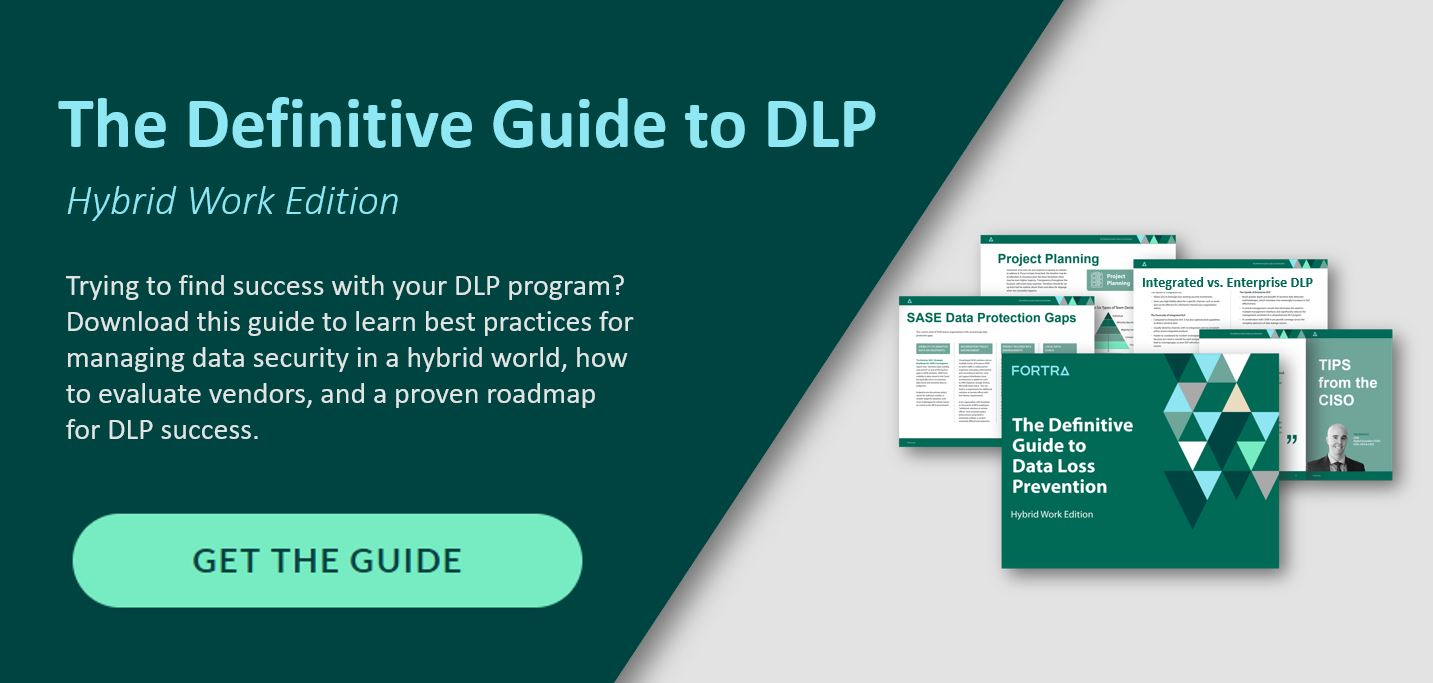
Additional DLP Resources
Want to learn more about data loss prevention? Here are some of our favorite DLP resources: